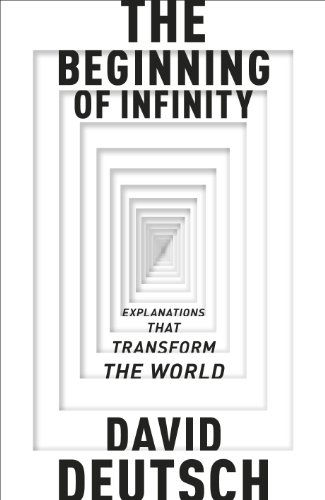
The Beginning of Infinity is an enlightening book by David Deutsch. It sheds light into the nature of scientific progress, which consists not of extrapolating past successes, but rather identifying unanswered questions and overcoming them.
The Black Swann Philosophy is perhaps a central tennet in Deutsch's book. Namely, concerning the successful theories which try to explain certain phenomena in the real world, we should not ask why are they good, but rather how are they bad and how can they change to become better.
In order to make progress in any scientific field, we have to ask new and harder problems each time. Because any solution given to these problems are theory-laden, to improve on theories we need new problems which guide new theories. The path to a good answer or theory is not straight, but rather bent. In searching for a good theory/explanation/answer to a problem, we may have to take a detour in identifying tangential problems that could, maybe luckily, help us answer an original question.
This has made me reflect on how we should make progress in AI. On one hand, we can gaze at the current successes in the history of AI and expand on deep learning.
Well, ever since the start of the 21st century, thanks to the increase of computational power and omni-presence of data, what used to be small neural networks that could only approximate Boolean logic or very small-scale digit recognition have scale up to large deep learning models who are achieving state-of-the art performances on several benchmarks meant to measure a certain faculty of human intelligence: human vision or NLP through QA. What empowers these results are the well-deserved research poured into deep learning with regards to exploring novel architectures, optimization techniques, et al. which can redefine the new state-of-the-art performance on several canon benchmarks. See for instance how interest on deep learning have increased six-fold over the past decade according to Standord’s AI index report on 2021.
From this trend of successes, one can ask: how can we go beyond current state-of-the-art performance? Successes in deep learning suggest that we can further extrapolate deep learning’s infrastructure, which should map to increases in functional performance. See an analysis done Kaplan et al., (2020) on neural language models, which showed that language modeling performance improved smoothly as the neural model's size, dataset size, and amount of compute used for training increased. We can refer to this idea of scaling deep neural networks as the scaling hypothesis:
However, careful observers have noted that there are some very problematic caveats to the scaling hypothesis. It’s the (ignored) elephant of in the room ; namely:
1. brittleness due to inability for robust generalization beyond the training set distribution,
2. lack of explainability tied to the lack of computational semantics,
3. and lack of parsimony due to this evident over-reliance to big data, and unacceptable levels of computational power consumption as argued by Garcez & Lamb, (2020)
4. misleading benchmarks. For example, ImageNet contain biases such as object-centric bias, i.e. images tend to show the object to be classified centred, without being rotated, well-illuminated, and without confounding objects (Barbu et al., 2019)
Back to the original question, it can perhaps be reframed as: what something novel, what paradigm shift, rather than replicating our datasets, can we try to go beyond?
Comments