L∞p framework: Navigating through the muddy waters of the era of Artificial Intelligence
- Xuelong An Wang
- Jan 12, 2023
- 16 min read
As a fellow peer who is interested in embarking in the science of cognition, the adventure to learn AI has been, is and will be a path full of challenges, much akin to sailors looking for a treasure: they’re bound to navigate through tumultuous waters, subject to countless riddles and having to overcome a plethora of obstacles. Because interest revolving AI is overflowing, research, educational resources and/or societal discussion concerning AI is mirroring this trend, as evidenced from the growth in its research output (Savage, 2020), emergence of debates on its moral implications or massive open online courses devoted on teaching them (see Coursera, Udemy, edX, among others). As an interested learner, it is sometimes easy to get overwhelmed both by the pace in which knowledge grows and diversifies, and is thus a challenging feat to assimilate, that is, to make sense out of this complicated field. Naturally, one would attempt in organizing such knowledge with the hope of obtaining a clearer, neater view of the general landscape of AI, which is both useful for getting a sense of how eclectic knowledge geared towards the understanding of Human Intelligence is interrelated to one another (Figure 1), and subsequently get a sense of where we are in terms of the research, as well what’s still missing.

Figure 1 Cognitive Science schemata in the form of a hexagonal gem denoting the interrelationship between fields underlying the study of cognition. Although Artificial Intelligence is usually portrayed in societal discourse as an engineering problem, it can also better be viewed as a constituent field of a greater quest geared at understanding cognition. Image extracted from https://www.bb-learningsolutions.com/what-is-cognitive-science-2/
As a matter of personal interest, have you felt overwhelmed too? Just to provide a sneak-peak of what I’m referring to, consider the following: are Prof. Geoffrey Hinton’s capsule networks related fundamentally to the plethora of research concerning visual cortex, e.g., the Thousand Brain’s Theory by Jeff Dawkins or Karl Friston’s predictive coding theory. That is on the subject of vision, what about how it relates to language? Where does Chomsky’s Universal Grammar fit in or whether it should fit, whether the probabilistic models of recurrent neural networks and transformers can unlock the mysteries of human language. There are also tangent movements such as neurosymbolism with protagonists such as Prof. Joshua Tenenbaum who seek to marry the ideas of learning and reasoning by combining their strengths hoping both paradigms can mitigate the weaknesses of both such as brittleness, and black boxes. Even more, there is reinforcement learning and what are its implications on our understanding of intelligence. This reminds me of Prof. Song-Zhu Chun, which advocated that we are stepping into an area of unification, where diverse disciplines of AI (vision, language, prediction, gaming) should find their converging ground and devise unifying algorithms that can consider all these faculties, a realm known as the dark matter of intelligence or simply dark learning. Up until now, we’ve been talking about algorithms only. Algorithms run on physical substrates, thus in the context of cognition, the brain shouldn’t be excluded. Is the brain the product of engineering or evolution; where does the selfish gene fit in, and how are discoveries in the biological, neuroscientific realm related to the quest to achieve artificial intelligence on computers…
Given this torrential influx of knowledge, questions such as how are they inter-related to one another are important for us in getting a neater, intelligible view of where the field of AI is positioned and where can it be headed. In general, knowledge derived from a diverse range of fields should not be viewed as isolated islets, but rather as constituent elements of the same underlying framework to understanding Human Intelligence. Hence, an issue this article tries to address is the design of such framework that helps organize and accommodate such eclectic, seemingly independent pieces of knowledge. Notice though that these concerns of organizing knowledge do not involve industrial or technological aspirations (yet) given that we are not forming judgments about the successes and failures of the implications of discovering such diverse knowledge. Rather, we are currently interested in how they are related to one another, as well as ask whether they can be neatly organized to provide any insight relevant to the mind.
Topologically, there are diverse ways to organize all the knowledge we’ve been learning through manifold sources centred on the topic of AI. One such example would be through mind maps, of which advantages is that it can delineate concepts and establish the relationship amongst them (see Figure 2).
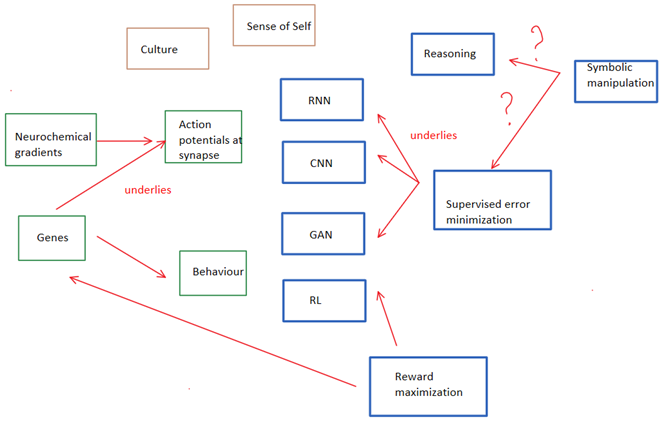
Figure 2 An example of a mind map organizing knowledge derived from the research on Human Intelligence. Because this mind map is simply meant as an example of a good but insufficient framework to organize knowledge, it has been kept brief. For an in-depth insight into each concept outlined above, consider referring to MOOCs in Coursera, (Adate et al., 2020) and/or (Richard Dawkins, 1976) . Boxes with the same colour outlines are preferably clustered together given their commonalities. Namely, what is inside green boxes mainly refer to physical substrates or observable phenomena that is instantiated in the real world; to put it simply they are present in the tri-dimensional space. What is in blue, however, are mostly algorithms and models devised at performing a human faculty of learning and reasoning and are thus bi-dimensional concepts. These models are designed with the main purpose to understand the environment. Some proponents have suggested that models should do reasoning over through input symbols. Other have designed supervised methods to either discriminate elements of the environment or learn the parameters of latent probability distributions that could generate environmental data . Alternative methods include unsupervised ones which simply cluster qualitatively similar data in the environment. Red arrows with a question mark denote an active contemporary query on whether symbolic reasoning and probabilistic learning are the same or differing concepts. In brown boxes are concepts which are hard to cluster to either of the above; for instance, culture may both refer to tangible objects or practices reminiscent of certain tradition or of historical significance, but may also refer to abstract belief systems or doctrines.
However, there are concepts that can be clustered together or that demarcating boundaries are not as explicit as one would expect: an example of such would be that no matter deep learning models are optimized through gradient descent, with optimization techniques which are meant to improve on the quality of such without tweaking the underlying principle of minimizing a loss function. In other words, regardless of whether it’s a vision-, language-based, model, it would be neater to cluster them together given that they’re currently running on deep-learning paradigm of stochastic prediction-error minimization. Another additional issue is that mind maps can become “messy” and prone to confusion after one tries to revisit them or tries to update them with emerging knowledge.
An alternative topology that would both inherit the above property of demarcating contrasting concepts, as well as be able to cluster together similar concepts could be the Venn diagram. It can also identify concepts with overlapping properties along the way (Figure 3).
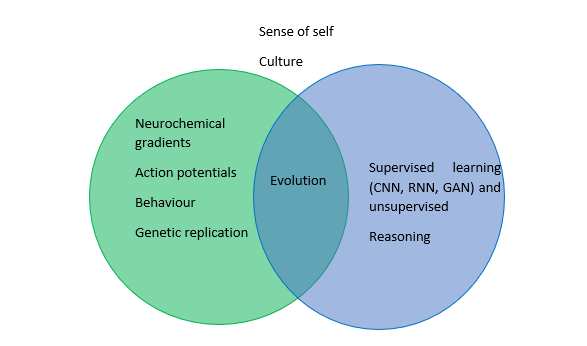
Figure 3: Venn diagram which mirrors the mind map of Figure 2. The colour code above has been maintained, in that green represents findings concerning the tangible aspects of Human Cognition as expressed in some forms of neural activity or behaviour, while blue represents proposed models and algorithms which shed some light to the darkness of Human Intelligence. An intersecting property can also be identified, which is that of evolution as present in both sides of the Venn diagram.
Under this framework, we can identify the advantages of how it organizes knowledge by seeing it as an extended capability with respect to Figure 2, since it is able to cluster together qualitatively similar knowledge. We can accommodate neuroscientific or psychological findings concerning brain activity or behaviour respectively and contrast it with research findings from the field of AI with proposed models such as those mentioned in Figure 2. The Venn diagram is also useful given that it allows us to identify a common denominator amongst findings from the different fields of study revolving around Human Intelligence, namely evolution, which refers to an iterative process which satisfies the optimization of something. In an anthropological or biological context, evolution is present in the development of the gene as a replicating unit of life, while on an AI or computer science context, although not called as evolution, there still exists an iterative process by which certain optimization problem is designed to be solved, whether it is error minimization in the supervised learning paradigm or reward maximization in RL. Once again, however, the challenges of findings revolving culture or sense of self is difficult to be accommodated on such framework given that there are inherent imprecisions on defining them, as well as research that is inherently contradictory revolving these concepts (consider this example, while it is undisputable that the self is “something” intangible and internal in each of us, recent arguments defend the idea that the boundaries between certain tangible objects of the environment and the “I” are very blurry, see (Clark & Chalmers, 2000) or (Clark, 2002).
The Venn diagram is useful in neatly organizing such diverse knowledge revolving around AI. Nonetheless, we can go a step beyond a schema which accommodates contrasting knowledge along with some intersecting concepts. That is, what if we take a step back and ask, are research findings from the different areas in Figure 1 doomed to be solely different to one another? How can a schema which highlights differences amongst diverse knowledge also denote the inter-relationship between one another? How can we represent the idea that although the eclectic knowledge above is qualitatively different, there still exists an underlying unifying framework that denotes their inter-dependence? An alternative schema proposed in this article is the ancient symbol of Yin-Yang and is used to accommodate the above diversely derived knowledge under a unifying framework. We can first look at some extended properties that are built on top of the schemas presented above (Figure 4).

Figure 4 Properties of the Yin-Yang schema which are helpful for accommodating all the research findings or knowledge revolving around Human Intelligence. It can cluster together qualitatively different concepts as in Figure 1 and 2, following a colour code where white corresponds to green and black to blue respectively. Additionally, it can also denote the complementarity of findings derived from the tangible world, as well as models and algorithms proposed to parse the environment. Intersecting properties of eclectic knowledge can be captured by the duplicity of the black and white portions in the light and dark sections of the symbol. Complementarity is represented by how both sides fit with one another, which is more expressive than just intersecting each with one another because apart from representing the idea that two properties may share a common denominator, complementarity also denotes that each portion can be integrated with one another to form a whole just like pieces of a jigsaw puzzle.
As important addition by the Yin-Yang framework is the notion of integration of parts. Namely, knowledge derived from fields of study such as neuroscience or anthropology which deal with the tangible world should not only be seen as different to research findings concerning the intangible world (models and algorithms), but rather complementary to them.
An interpretation that can be derived from accommodating the above knowledge within the Yin-Yang framework is that we can distinguish at least distinguish three levels of research concerning Human Intelligence: on one level, the white portion Yang, there is research revolving around the tangible, natural world where the protagonist being studied is the non-human animal. Because of its observable behaviour that is open to empirical examination and experimentation, it is allocated to the transparent side of the Yin-Yang framework. At a more fundamental level, the Yang is comprised of the unit of life: the selfish gene. The main purpose of the gene is to replicate, which driven by the motors of evolution across billions of years since Earth welcomed its first primaeval beings led to the emergence of organisms which can display complex behaviour (selfishness, altruism, organization, et. al) centred on ensuring longevity, reproducibility copying fidelity, i.e., to live as long as it can, to reproduce as much as possible and that such replicas are as similar to the ancestor.
On another level, the Yin portion, we can allocate research revolving around intangible algorithms that are meant to understand the natural world. The protagonist in the Yin portion is the number, the basic unit of math from which models are built upon. Given that the Yin mostly tries to address the question of how to build abstractions of the environment, and is therefore intangible or unobservable, it is suited to this opaque portion (this is, interestingly, reminiscent of the concept of neural networks being “black boxes” in that we know what inputs can be mapped to outputs without understanding the whydunit, i.e., the intermediate process). Research concerning how to build such neural network models of the environment is less mature compared to research derived from the Yang portion, so it is debatable what fundamental principles lurk on this side. Because there is still a long, arduous journey on the inquiry of how rich representations can be formed from the surrounding world, what is said as follows is subject to imprecisions and debate. A lot of research and successes in artificial intelligence suggest that, at a fundamental level, a model’s capabilities should at least include the ability to learn higher level, generalizable abstractions (make analogies) from input data, do logical reasoning from it so as to identify problems with the data and form hypothesis on how to solve them and also have the capability of introspection to optimize these calculations.
Finally, on the uppermost level is the integration of both Yin-Yang, that is the result of complementing the biological principle of replication through interacting with the environment and the mathematical principle of modelling the environment through building an abstraction from it. It is proposed that the result of such integration, which may have occurred at some unknown time in the distant past over our course of evolution, gives rise to the complexities of what makes us human: consciousness, emotions, creativity, et. al, can be thought as the result of the interplay of basic principles headed by a selfish gene and imaginary number (or perceptron) (Figure 5). In this integration, the whole is greater than "the sum of its individual parts”. However, the in-depth development of each individual component can’t lead to the emergence of the whole. In other words, without attempting to integrate the principles of each Yin and Yang, but instead trying to simulate only the principles of a singular component may not lead to the emergence of all possible functionalities of a human faculty and is also vulnerable to anthropomorphic biases. For example, a non-human animal who can follow Human Language instructions should not be interpreted as a reflection of “understanding” what the instruction entails, but rather a classical case of reward-signal pairing. It is argued that with similar scepticism we should view, for instance, mechanical models which emulate Human Language given that they mostly miss the principles of the Yang portion which include communicated based on the goals of reproduction or survival.
Given that a lot of faculties we consider inherently human such as intelligence, vision or language exist at least at 3 different levels: one inherent to nonhuman animals (Yang), one to machines (Yin) and another to humans (Yin-Yang). In order to distinguish them, I’ve been careful in referring to human faculties with uppercase letters, as in Human Vision, Human Language and Human Intelligence as qualitatively distinctive to vision, language and intelligence achievable by either non-human animals and non-human machines.
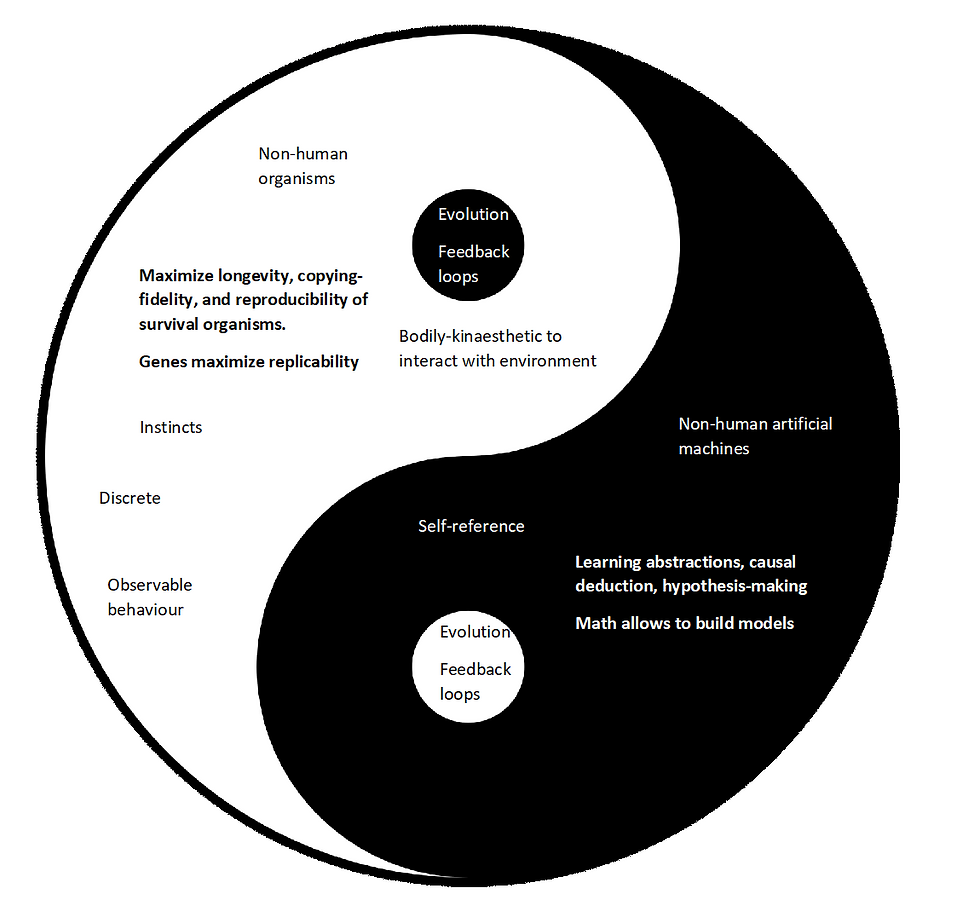
Figure 5 The loop framework, where multi-disciplinary knowledge derived from the path in understanding Human Intelligence is accommodated in an attempt to organize it neatly. We can distinguish research findings that focus exclusively on non-human animals on one level, on non-human machines on another and on humans at an upper level. It is argued in this article that it is a more suitable framework than the Venn diagram or mind maps in accommodating the above knowledge. For brevity, basic concepts and principles have been allocated on each side, where the colour code mentioned in Figure 4 is followed. Importantly, throughout the article I have been careful in employing uppercase letters (as in Human Intelligence and Human Language) to denote capabilities achievable not by either individual components Yin or Yang, but rather only attainable through the integration of principles denoted by both. In other words, it is proposed that non-human animals can at most achieve certain form of intelligence or language (notice the lower-case initial letters), and this is the same for non-human machines. Specifically, non-human animals can at most have visual, bodily-kinaesthetic, and its own linguistic intelligence for inter-species communication which aid their survival and reproduction, but not enough to model the environment and understand it as humans do, such as being creative or make hypothesis. Parallelly, given the trajectory of artificial intelligence, it suggests that the capabilities of artificial machines (the Yin portion) can only develop logical-mathematical, visual-spatial, introspective and its own form of linguistic intelligence to perform reasoning and inter-machine communication, but it is qualitatively under-developed compared to that of human, such as being unable to manipulate the real world and cause significant changes useful to perform science and technology. This is unless there are biological principles of replication integrated, since then the artificial machine may be required to have a body and develop bodily-kinaesthetic intelligence.
Upon first glance, the Yin-Yang framework can be reminiscent of Descartes’s mind-body duality given that the mind is abstract, and the body is tangible. However, this is not necessarily the case. First, the Yin-Yang is preferably not dedicated to denoting duality but rather complementarity in the sense that although the gene and perceptron are different to one another, they can cooperate to build survival-based, Intelligent beings. Second, it is proposed that the mind, being a faculty exclusive to humans, is the result of the Yin-Yang integrated, i.e., neither individual component (materialized as non-human animals in the Yin or non-human machines in the Yang) can acquire the mind because of the inherent limitations of each individual component. For example, as mentioned in Figure 5, while non-human animals can be said to have some degree of understanding of the environment such as to do basic perceptual discrimination serving the interests of survival or reproduction, this capability can’t mirror that of humans who can perceive not only the explicit environment, but also implicit meaning and abstractions from it. Finally, the mind is not necessarily different to the body, but is rather spread over it, in a phenomenon known as Embodied Cognition (please see Wilson & Foglia, 2011) and even the Extended Mind (Clark & Chalmers, 2000). Therefore, once again, the Yin-Yang framework testifies not the duality of mind-body, but rather that the integration of Yin-Yang yields the mind which mainly resides in the brain but is spread across the body.
Looking back at Figure 5, the Yang represents organisms which are mainly concerned with survival and reproduction and can do some degree of computation geared to these purposes, while the Yin are artificial machines which are mainly concerned with performing computations on parsing the environment and to a lesser extent interact with the environment. Artificial machines are limited in that they can’t act upon the physical components in which they are made of so as to replicate them, nor influence the surrounding environment to a similar extent as gene-based survival machines can. The medium, or the corpus, of artificial machines are more rigid and constrained physically; however, they are very flexible in the mathematical abstractions they can theoretically achieve. This contrasts with the Yin portion which are made up of very flexible components (genes) which can store some form of basic computation which guarantee the fulfilment of the purpose of replication and has done so at an evolutionary timescale. We can think of humans being the result of such properties where we can observe the close to infinite reach of the abstractions achievable by the mind, as denoted by creativity, self-reference, Human Intelligence and Human Language. It’s partly computation which rests in a physical substrate mainly concentrated in the brain but also spread throughout the body (embodied cognition), and our body display flexible properties of the Yang as denoted by our abilities of reproduction, self-repair, organic consumption, metabolism, and death. This kind of integration of Yin-Yang is beyond a simple combination of two components in the sense that the whole (Yin-Yang) is greater than the sum of its individual components (Yin or Yang), given that the whole can exhibit properties unattainable by neither of each. For instance, no matter how agile animals can be, the computations they can perform is limited and as such can’t develop the same degree of curiosity as humans do in seeking to understand the world. Also, Humans in general are, biologically speaking, survival machines, yet on top of this evolutionary substrate the ability to store information and to perform highly complex computation on it was developed to the point that it is to some extent dependent on it but can also exist independently. For instance, we humans can wonder on the mysteries of the universe beyond being subservient to our genetic desires of sexual reproduction, yet paradoxically highly dependent on it to self-sustain our biological bodies through organic consumption. Despite its independence, the gene is also highly reliant to the mind for a more efficient spreading given that the capacities of the mind, the ability to achieve technology and dominate the natural world, has given it the undisputable position of the dominant animal in the biosphere, thus ensuring its selfish spread for the indefinite future. This kind of self-fulfilment of needs, that’s paradoxically paired with inter-dependence, which again is restored to inter-independence, testifies the underlying loop which unifies the basic properties of understanding through modelling the environment and surviving to reproduce in it. Hence, the name given to the framework is “loop”; it takes two principles to loop into the highly complex human being.
Another interesting advantage of the loop framework is that it allows to question the limits of either the Yin and Yang individually, and gear us to ask how to overcome those limits, which is relevant for the less well-understood Yin portion which deal mainly with finding how to build models that can parse the environment. Conversely, had the limits been not explicitly stated and reflect on the complementary Yang portion, we may get over-hyped by the current successful models that are meant to emulate human faculties such as vision and language, and subsequently choose to dive deeper into current solutions rather than think of different ways. However, it is possible that elaborating deeper into a single component would not lead to the emergence of the whole.
In conclusion, through this article it is hoped that that the loop framework can help mitigate the torrential inflow of multidisciplinary knowledge produced in the quest to understand Human Intelligence. Hopefully, it can help us look at the work of AI in a clearer, more organized way; that is, to understand it as, at least, the voyage in understanding the principles of replicability of components and the capability for abstraction by those components, as well as how to integrate them. With that in mind, I can only wish you happy learning, as well as keep calm and don’t be overwhelmed!
42
42
42
References
Adate, A., Arya, D., Shaha, A., & Tripathy, B. K. (2020). 4 Impact of Deep Neural Learning on Artificial Intelligence Research. Deep Learning, 69–84. https://doi.org/10.1515/9783110670905-004
AI Podcasts. (n.d.). AI Podcasts. Lex Fridman Podcast; YouTube. https://lexfridman.com/podcast/
The plethora of AI Podcasts between Lex Fridman and amazing rsearches such as Daniel Kahneman, Melanie Mitchell, Noam Chomsky, Roger Penrose, et al.
Clark, A. (2002). Natural-born cyborgs : minds, technologies, and the future of human intelligence. Oxford Univ. Press.
Clark, A., & Chalmers, D. (2000). THE EXTENDED MIND. https://www.nyu.edu/gsas/dept/philo/courses/concepts/clark.html
Deutsch, D. (2011). Chapter 5: The Reality of Abstractions. In The Beginning of Infinity : Explanations that Transform The World. Penguin.
Hawkins, J. (2021). A Thousand Brains: A New Theory of Intelligence. Basic Books.
Hofstadter, D. R. (2008). I am a strange loop. Basic Books.
Ng, A. (n.d.). Deep learning specialization. Deeplearning.ai - Coursera. https://www.coursera.org/specializations/deep-learning
Richard Dawkins. (1976a). The Selfish Gene. Oxford University Press.
Richard Dawkins. (1976b). The Selfish Gene. Oxford University Press.
Savage, N. (2020). The race to the top among the world’s leaders in artificial intelligence. Nature, 588(7837), S102–S104. https://doi.org/10.1038/d41586-020-03409-8
Song-Chun, Z. (2017). Qiantan rengongzhineng: xianzhuang, renwu, goujia yu tongyi [AI: The Era of Big Integration Unifying Disciplines within Artificial Intelligence]. Shijiao Qiusuo. English version at: https://dm.ai/ebook/
Wilson, R. A., & Foglia, L. (2011). Embodied Cognition (Stanford Encyclopedia of Philosophy). Stanford.edu. https://plato.stanford.edu/entries/embodied-cognition/
Comments