Overview Part 1.0: Keeping up with the pace of technological advancements.
- Xuelong An Wang
- May 30, 2020
- 6 min read
“It is not the strongest or the smartest who survive, but the one most adaptable to change”
Charles Darwin
As Prof. Darwin once shared with this world, those that live to witness the continuity of history are not necessarily the strongest or the smartest, but the one that can readily adapt to the changes of environment. As for this teaching, it may well not only be targeted at humans only, but also to technologies too.
Throughout the course of history, new technologies (be it in the form of innovative techniques, products or services) have constantly substituted their predecessors. For instance, thanks to the development of the steam engine, afterwards the internal combustion engine, followed by the implementation of gas turbines and electric motors, allowed humankind the leave behind the use of horse carriages as means of transport and instead employ airplanes, high speed rails, cars, steamships (Kai-fu & Wang, May, 2017) to communicate with and across the rest of the world. The transport industry keeps upgrading itself, and in the near future, motor vehicles may just be replaced by electric driverless cars, marking another step of innovation in the history of mankind.
Another story to share: in the early stages of the development of artificial intelligence, a rules-based approach was used, that is hand coding a set of specific task-oriented algorithms for the system to solve a problem, so for instance, natural language processing was a task performed through implementing a set of grammar rules. This approach was then gradually substituted by an upgrade in learning techniques for computers: machine learning (for the above case, instead using a statistical approach to analyze large corpora of texts to extract rules), where generic algorithms that could resemble human learning were preferred for machines to become robust when designed to solve certain task. This technique then upgraded itself using neural networks, from which a plethora of novel techniques stemmed: deep neural networks, generative adversarial networks[1], convolutional neural networks, among others. These networks tend to require an enormous amount of training data to be able to extract relevant features and adjust the necessary parameters to perform small tasks such as image recognition, language translation or speech processing. Such procedure could be called “big data for small tasks”; nonetheless, it can be further innovated as it doesn’t resemble biological neural network’s ability to be able to exploit “small data for big tasks”, such as predictive processing, abstract, higher dimension reasoning, creativity, among others. So the next step or the next upgrade to be pursued in the field of AI can be, as Prof. Song Chu term it, to inquire into the “dark matter” regarding human cognition and how it can be applied to AI (Zhu, 2017)[2]. As an example of such approach, by late 2017, Prof. Geoffrey Hinton, who is regarded as the creator of deep learning[3], proposed the concept of “Capsule Networks” as an alternative to Deep Learning that could perform better in image recognition or video processing with less data (Eric, 2019; MIT Technology Review, 2019).
The above anecdotes may differ in content, but an overall common denominator they share is the pursuit of a technological upgrade because such upgrade might be the one that’s suitable to adapt to the requirements of the current technological era, hence the pursuit or pressure for innovation is extrapolated. A field in which I want to pay particular attention is in medicine, specifically in diagnosis as well as therapy of diverse diseases and how AI can merge with it in order to improve the accuracy rate of diagnosis as well as develop targeted therapy. There are already notable achievements in this hybrid field, such as neural networks trained on medical imaging that can outperform radiologists, ophthalmologists, dermatologists; NN trained on electrocardiogram readings to predict the possible onset of a heart attack; NNs trained on genomic datasets; NLPs to act as a virtual psychotherapist; NLPs to automate the reading of research papers; virtual records to constantly track the gut microbiome based on food intake to adequately balance the amount of nutrients to take; among others. Of course, these advancements come along with their respective drawbacks, such as research and applications is not equilibrated yet, some fields deserve further understanding as well as employ larger and more diverse datasets (Eric, 2019)[4].
However, most research regarding diagnosis as well as therapies of diseases tend to focus on the phenotype related to a disease, that is, what are the physical, observable symptoms of a patient, i.e., form of a skin rash, certain tumor’s size, symptoms such as cough, chronic distress, inflammation, pain, among others. So, for example, medical imaging seems to rely heavily on recognition of features in a lung scan or features of a skin rash to make an accurate diagnosis.
An upgrade, therefore, concerns the exploitation of information carried by the genotype related to a disease and, indeed, currently, we are seeing great advances and focus on the research of the genome related to a disease, diving into the realms of genomics and epigenetics to study disease and manufacture possible therapies. This area of study spans from genetic sequencing[5] to identification of genetic biomarkers of a concerning disease, as well as genetic engineering for the development of targeted therapy for that particular disease.
Given the above introduction, I hope I’ve convinced you of the importance of identifying what are the current technological upgrades in the diverse fields of study such as AI. We can ponder on ourselves: what technologies will keep up with the fast pace of development and replace their predecessor, akin to how only adaptable species nowadays remain? How can we, as stargazers of the future, protagonists of the incoming generation do and act in order get ahold of those technologies and catch up with these advances and development, to keep up with the current flow of history, a swirling torrent?
In medicine, I believe that it’s diving into the realms of genomics for early diagnosis and targeted therapy of a disease, and the biomarker presented here in the articles to follow is called microRNA and how it might play a crucial role in the development for an upgrade in this field.
For a brief sneak peak of what might the ripening of the study of how microRNA can impact the future of medicine, here is a hypothetical dialogue:
Doctor (calls): Hey Edmund, from the blood sample you provided yesterday and processed through the mirOculus at your home, I can see that the results show an upregulation of microRNA-122 that suggests you are developing Hepatitis C.
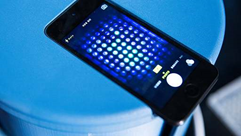
Edmund: What? Doc I’m so worried.
Doctor: Don’t be worried, you can come to the hospital and we can perform a subcutaneous injection consisting of oligonucleotides that can inhibit the upregulation of that microRNA. This should disrupt the progression of the disease and provide long lasting antiviral activity. Don’t forget to constantly monitor the microRNA pattern of you and your family.
Edmund: Okay, thanks Doc, that sounded…quiet impressive….
For those gazing at the future, I present microRNAs, a molecule which might become the next blockbuster in the era of the merging of medicine and AI by playing crucial roles in the early diagnosis as well as targeted therapy for diverse diseases. Of course, I have to clarify that microRNA targeting is just one of a myriad of innovative technologies to keep an eye one, analogous to only one boat from a crew that can carry us, young passengers, through the turbulent tides of technological revolutions that can only move forward.
Bibliography
Clark, A. (2013). Whatever next? Predictive brains,situated agents, and the future of cognitive science. BEHAVIORAL AND BRAIN SCIENCES, 1-7, 20-21.
Eric, T. (2019). Deep Medicine: How Artificial Intelligence Can Make Healthcare Human Again.New York: Hachette Book Group.
Kai-fu, L., & Wang, Y. (May, 2017). 人工智能 (Artificial Intelligence). Hong Kong: 文化发展出版社 (CHINA CULTURE DEVELOPMENT PRESS).
Seth, A. (2017, July). Your brain hallucinates your conscious reality. Retrieved from TED Ideas worth sharing: https://www.ted.com/talks/anil_seth_your_brain_hallucinates_your_conscious_reality
Soto, J. (2014, Oct). The future of early cancer detection? Retrieved May 2020, from TED Ideas worth spreading: https://www.ted.com/talks/jorge_soto_the_future_of_early_cancer_detection
Xiong, C., Shukla, N., Xiong, W., & Zhu, S.-C. (2016). Robot Learning with a Spatial, Temporal, and Causal And-Or Graph. IEEE Robotics and Automation Letters (RA-L), 1-4, 8.
Zhu, S.-C. (2017, 11 02). A light discussion of Artificial Intelligence: Current Situation, Purpose, Framework, and Unification | Finding the root of the issue (浅谈人工智能:现状、任务、构架与统一正本清源). Retrieved from Untitled: http://www.stat.ucla.edu/~sczhu/Blog_articles/%E6%B5%85%E8%B0%88%E4%BA%BA%E5%B7%A5%E6%99%BA%E8%83%BD.pdf
[1] For further inquiry into these networks, the following website for learning can be visited: https://distill.pub/ [2] The cited source is the original document in Chinese published by Prof. Zhu. For an English version in the format of an e-book, please visit: https://dm.ai/ebook/ [3] Along with Yoshua Bengio and Yann LeCun. [4] For further exploration on such topic, I recommend reading Deep Medicine by Dr. Eric Topol. [5] Please see The Human Genome Project: https://www.genome.gov/human-genome-project
Comments