Part II: DeepMiRNA: miRNAs as biomarkers for early diagnosis of diseases
- Xuelong An Wang
- Sep 2, 2020
- 16 min read
“The best cure for any disease is its prevention”. A more down to earth paraphrasing can be the “best cure for a disease such as cancer and its diverse presentations like leukemia, thyroid carcinoma or other disorders like neurological ones such as Alzheimer, is their early diagnosis and subsequent targeted/personalized therapy. For the former, current approaches to achieve an early diagnosis for the diseases above mentioned are not as effective as expectations would demand. They mostly focus on the phenotype expressed by the disease, such as lung tumor size (lung cancer), skin rashes (skin cancer), identify the presence of protein complexes like beta - amyloid, that come with its disadvantages such as the difficulty to at a premature or early stage identify a primary cancer (which can be as low as 100000 cells undergoing mutations). Current approaches that involve artificial neural networks trained at image recognition in order to identify at an early stage features of diseases like cancer from radiographies has shown promising results by outperforming real ophthalmologists, radiologists and pulmonologists; of course, these innovative approaches hasn’t yet to meet a large scale real-world applications, and in practice they meet their limitations such as the data gathered such as electronic health records being stored in different formats so it can’t run on diverse software or not having a diverse enough dataset that’s able to capture health tendencies among distinct ethnicities (Eric, 2019). Also, when diagnosing for disease like Alzheimer or colorectal cancer, methods are too invasive and excessively technical; for the former, an early diagnosis with a time window of about 5 years requires the extraction of cerebrospinal fluid to measure the amount of beta – amyloid proteins, and for the latter, colonoscopy which as mentioned might struggle to identify a primary cancer that may begin with as low as 100000 mutated cells (Esther, 2020). Affordability, accessibility and availability become concerning issues too.
Given the described issues, the need to find novel, upgraded ways to deliver medicine is thus necessitated; in this case, it’s reinstated the an idea shared previously, one can now also focus on the genotype related to a disease.
Around 20 years since the creation of The Human Genome Project, the study of genomes has attracted researchers thanks to the seemingly causal relationship shared between pathogenesis and genetic makeup. Furthermore, as more understanding is built upon from the Human Genome, attention is shifted from protein coding sections to the nonprotein-coding RNAs, a field which have vastly expanded with ongoing research studies and now include transcripts such as Piwi-interacting RNA (piRNAs), long ncRNAs (lncRNAs) and microRNAs (miRNAs), the last one arguably are one of the most predominantly represented ncRNA groups in clinical research (Hanna, Hossain, & Kocerha, 2019).
The field of study of miRNA, which has acquired for itself the name of “miRNomics”[1], has attracted much attention due to it being yet another potential candidate as a biomarker for the prognosis or diagnosis of several diseases, which include: diverse cancers like thyroid carcinoma (Celan, et al., 2017), neurological disorders like Alzheimer (Cheng, et al., 2015), liver diseases like hepatitis B, even infectious diseases like tuberculosis (Naveed, et al., 2018), as well as other cardiovascular, skin or skeletal muscle diseases (Ardekani & Naeini, 2010), among others.
In contrast to diagnostic methods that may be invasive like biopsy or require taking samples that are not easily accessible like cerebrospinal fluid, miRNAs appear to be easily accessible given that they may circulate in extracellular vesicles called exosomes of the blood (Hanna, Hossain, & Kocerha, 2019), or may be released passively into the bloodstream from dead cells; they are also mostly organ, tissue or even cell specific (Ming, et al., 2016), which may open the opportunity that a blood sample can reveal a particular up/down regulated expression pattern that may pinpoint which section (be it organ or tissue) of the body is undergoing pathogenesis.
Even diseases like Alzheimer seems to be able to be prognosticated through a miRNA signature detection despite being a neurodegenerative disorder which current diagnostic methods involve extraction of cerebrospinal fluid, for instance. This is because miRNAs are able to cross the blood-brain barrier, therefore can potentially be quantitated in routine blood, serum, or plasma tests as measures for, not only Alzheimer, but other neurodegenerative and neurodevelopmental impairments (Hanna, Hossain, & Kocerha, 2019).
As an additional point, it’s worth reinstating that the potential for miRNA-based diagnosis is better exploited when prognosticating a disease, as an early detection increases a patient’s survival. As a matter of fact, regarding Alzheimer, it seems from (Cheng, et al., 2015) that miRNA biomarkers are not suitable to diagnose late-stage Alzheimer disease, but are better predictors during early- to mid-stage Alzheimer disease during beta-amyloid deposition.
miRNAs as potential biomarkers
The current literature mostly emanates an optimistic atmosphere regarding the measure of relative miRNA’s concentrations to prognose a disease. This is because several miRNAs have been discovered to be linked to the capability of regulating either cell proliferation, apoptosis, differentiation as well as diverse molecular pathways. Deregulation of these miRNAs (whether it’s upregulation or down-regulation) can lead to the development of a disease: examine for instance the handmade table containing the abstract of different papers that has identified a miRNA signature related to a disease:
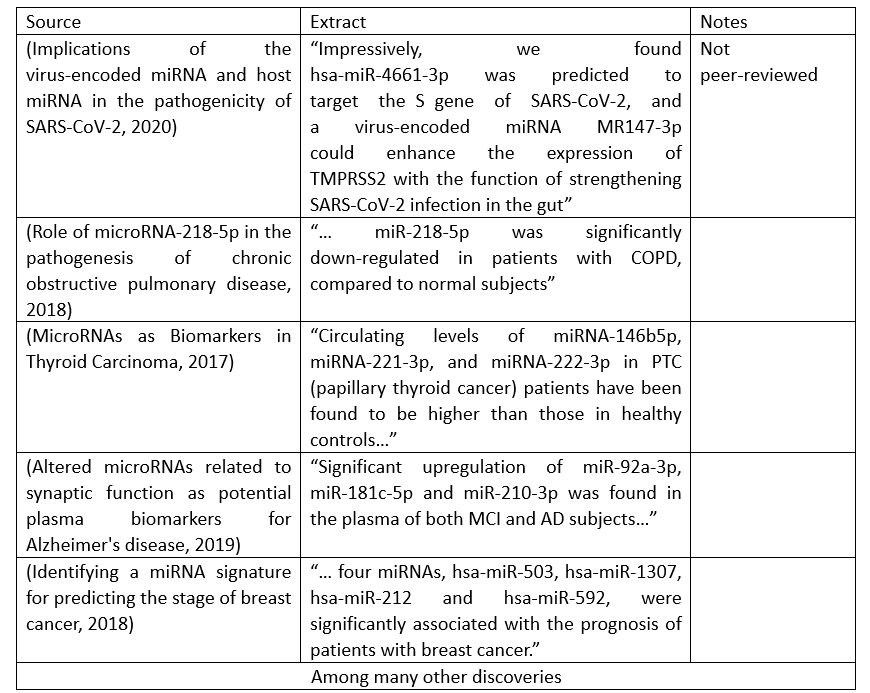
Table 1: Examples of discoveries that display the relationship shared between a group of miRNAs’ concentrations and its respective disease’s development; such discoveries can be employed for diagnostic and therapeutic purposes. The intention of sharing this table is to showcase the inability for a human being to keep up with the plethora of research papers being published that outline diverse miRNA signatures to prognose and/or treat certain disease.
Through diverse methodologies that combine experimental, bioinformatical or statistical analytical approaches, researchers are constantly identifying useful causal relationships between RNAs and the phenotype of a disease. However, because of the ever-increasing amount of research output, it’s becoming impossible for a human being to keep up with this large tide of discoveries; in the end, there are about 2000 miRNAs identified so far, and it is believed that they collectively regulate one third of the genes in the genome (Hammond, 2015). Additionally, it seems that not all miRNAs discovered should be necessarily treated equally as biomarkers or therapeutic targets. For instance, some cancer types seem to rely on fundamental oncogenic path-ways, so a core set of mature miRNA may exist that are consistently dysregulated across these cancers and drive pan-cancer tumorigenesis by recurrently targeting and dysregulating genes in these critical pathways (Ramkrishna, Clare, Jiang, Evan, & Christine, 2020). Also, another concerning issue is that past research has only focused on studying miRNA’s 5p strand, but as (Ramkrishna, Clare, Jiang, Evan, & Christine, 2020) noted, it seems that both 5p and 3p strands cooperate in the pathogenesis of diseases, opening yet another path for biomarker and therapeutic research. Not only is there an increasing amount of effort and research put into experimenting and results’ publishing, there might appear new paths that may contradict previous believes.
Despite such a dynamic, ever-evolving process of assimilation of discovered miRNAs, it appears that the fundamental task of identifying which miRNAs and how are they deregulated so that it causes the phenotypes that constitute the early development of a disease, remains unchanged. Due to this, this is where artificial intelligence can serve as a useful tool to aid reasearchers in extracting from the massively available papers relevant data about:
- How a miRNA’s up or down-regulation is related to the onset of a disease
- How that miRNA interacts with a biological pathway
- How that miRNA interacts with genes or other molecules such as another miRNA
- Among others
A viable approach is to train natural language processors to produce from inputs made up of research papers knowledge graphs that display the data about miRNAs laid out above.
Such an idea has been employed by startup company miROculus (previously mentioned, which focuses on detecting circulating miRNAs’ concentrations to achieve an early diagnosis of cancer) is displayed in Figure 1:
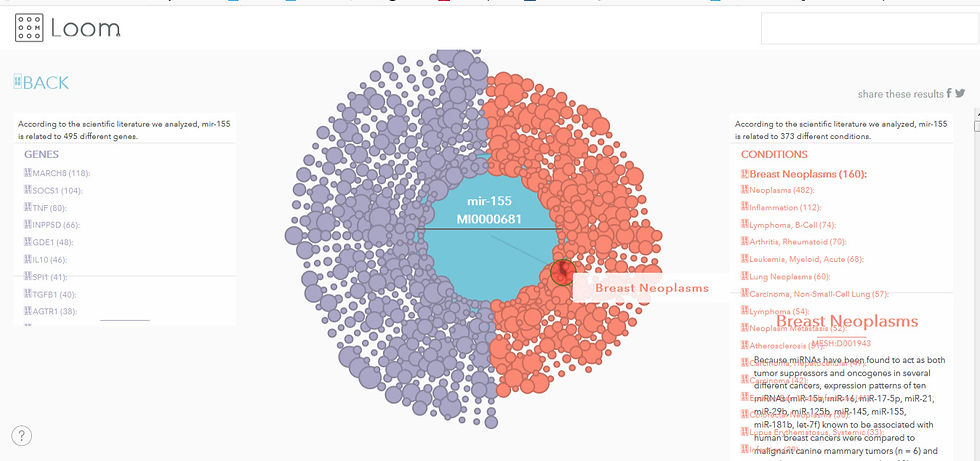
Figure 1: Screenshot of a graph authored by miROculus that displays the relationship shared between miRNAs such as miR – 155, genes targeted and diseases such as breast neoplasma
Source: https://loom.miroculus.com/#
According to a case study from Neo4j (a graph database), the miROculus team gathered more than a billion articles in Hadoop and used a NLP to extract specific sentences with keywords for gene, disease and microRNA and subsequently infer the relationship between keywords; the team developed an unsupervised machine learning model to classify relationships, which are then stored in Neo4j[2]. It’s also worth noting that it’s open-access tool for the science community, which opens the opportunity for research to support or refute any knowledge that’s displayed. Nonetheless, although it’s claimed that this interactive site is updated monthly (Courtney, 2017), the website itself displays its last updated date to be 2017, which is concerning as it may mean that it lags behind on new updates concerning miRNAs such as the 5p/3p strands cooperation.
Similar approaches that compile miRNA research results from papers have been done, such as online databases (please visit http://www.mirbase.org/ or http://www.cancerindex.org/geneweb/index.htm ).
Accessing miRNAs biomarkers
Of course, if we inquire deeply regarding the potential of miRNA as biomarkers, apart from sorting out the causal relationships, another concerning issue is the methodology employed to access and isolate them for clinical analysis, which methods may vary in precision or ways to perform it completely. For instance, Table 2 shows some methods used to isolate and analyze miRNAs from a biospecimen like blood plasma or serum:
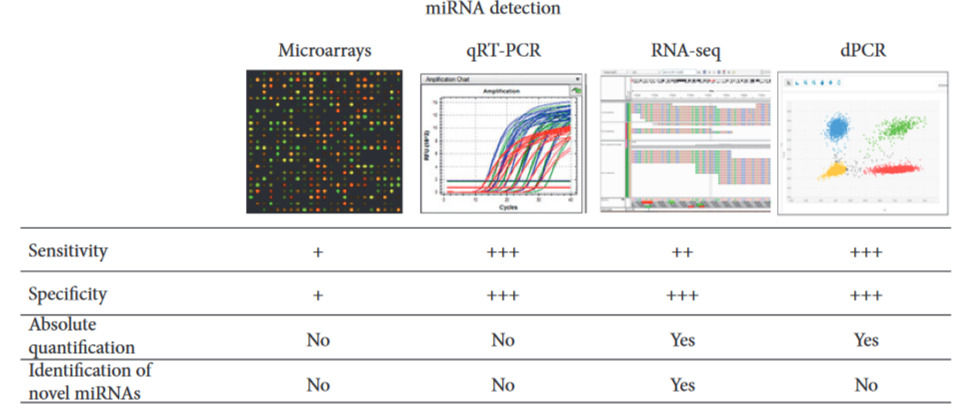
Table 2: Four methods used to measure the concentration of miRNA from a sample. 1) Microarray-based profiling allows rapid processing and its cost is relatively low. However, it is the least sensitive and least specific of the miRNA profiling methods, and it does not allow the identification of novel targets. 2) QRT-PCR is probably the most popular method currently used for miRNA detection. It entails reverse transcription of miRNA to cDNA, and it’s more specific and sensitive than microarrays. 3) RNA-seq is currently the most expensive technique for miRNA profiling, but it is also the most informative as it provides quantification data as well as sequence data which can therefore be used to identify novel miRNAs. 4) Digital PCR is superior to previously described methods in terms of sensitivity and precision, and it is the technique most widely used to study miRNA expression in plasma or serum samples. (Celan, et al., 2017)
However, although the above methods are able to complete the task of detecting and measuring miRNAs, they come with perhaps essential limitations: they require bulky and expensive equipment, intensive sample preparation, or long turnover times, which limit most of them to well-equipped laboratories (Richard, et al., 2019). Therefore, there is a pressing need to develop easy-to-use, portable, and amplification-free methods for miRNA detection.
Discussing about the simplicity of a method to detect and measure miRNA’s concentration is relevant as it influences whether it’ll be available to the general population or reserved to medical professionals or researchers. Issues to be taken into account are:
- Cost of reagents and tool: ideally, to keep them as minimum as possible if the goal is to “democratize medicine”, borrowing the words from miROculus co-founder Jorge Soto.
- Difficulty of methodology to process the (blood) sample and subsequent analysis: the ideal would be to design a method with limited sample preparation that a nonexpert could execute (Courtney, 2017), which would allow the capability to constantly monitor circulating miRNAs like how one would regularly track blood glucose and blood pressure from a glucose-meter and sphygmomanometer used at home. As such, the time taken to get the results of such measurements would also ideally be limited to within hours.
CRISPR-CAS System and miRNA diagnostics: search for a universal diagnostic method
CRISPR (short for Clustered Regularly Interspaced Short Palindromic Repeats) – CAS system was initially discovered in bacteria and archaea, as an acquired immune system against viruses and phages through CRISPR RNA (crRNA)-based DNA recognition and Cas nucleases-mediated DNA cleavage (Feng, Yan, & Xiong, 2014); hence why it was used in the domain of genome editing, gene therapy or transcription regulation.
However, recent developed techniques has also spanned to the field of nucleic acid detection. Such advancements have promoted next-generation diagnostics employing CRISPR-CAS systems such as Cas 12, 13, 14 by making use of their nonspecific degradation of non-target molecules (trans cleavage) after specific recognition of the desired nucleic acid (Yuanyue, Xiaoming, Ru, & Da, 2019). As an example of the application of the CRISPR – CAS 13a system, please see (Jonathan, et al., 2017), in which it’s shown how Cas 13a’s collateral promiscuous RNAse activity upon target recognition is exploited to detect strains of Zika and Dengue virus, distinguish pathogenic bacteria, genotype human DNA, and identify cell-free tumor DNA mutations[3].
Regarding miRNA detection, there also seems to be great potential yet to be discovered from employing the CRISPR-CAS 13a system. Unlike qRT – PCR, a Cas 13a based detecting platform doesn’t need to amplify the target molecule, as well as it’s relatively more low cost, easily scalable (doesn’t require much time nor technical steps) and it’s highly specific, meaning that it can discriminate highly homologous miRNAs from the same family. Such advantageous qualities are shown, for instance, from the research from the South China Normal University as well as from University of Freiburg.
For the former, (Yuanyue, Xiaoming, Ru, & Da, 2019) used a Cas 13a nuclease from Leptotrichia buccalis (LbuCas 13a) to form the CRISPR-LbuCas13a (Figure 2) platform that was tested on detecting miR-17. This detection system is very flexible thanks to the programmable crRNA and highly specific given that it can even discriminate single nucleotide variations among very homologous miRNAs. It’s also very simple to use thanks to the mild and constant reaction temperature and one-step detection (<30 min) it needs.

Figure 2: A depiction of how the CRISPR-Cas13a system detects a miRNA
Reporter RNAs are mixed with the sample with/out the target miRNA. If the Cas13a system’s programmed crRNA detects the desired miRNA from base complementariety, Cas13a’s cleavage mechanism is triggered which indiscriminately cleaves RNA nonspecifically, including the reporters, releasing fluorescence signals. Fluorescence signal, hence, will be proportional to the concentration of the target miRNA.
For the latter, (Richard, et al., 2019) designed a CRISPR/Cas13a-Powered electrochemical microfluidic biosensor (Embedded Video 1) for miRNA diagnostics, which was used to detect upregulated miR-19b in serum samples of children suffering from brain cancer. What’s worth noting is its readout time of 9 min and an overall process time of less than 4 h, a limit of detection of 10 pm while using a measuring volume of less than 0.6 μL. However, it seems that the system is not as highly specific given that it can’t discriminate miR-19b from miR-19a which has a single base mismatch, although the authors mention that such exact distinction is not compulsory as both miRNAs belong to the miR-17–92 cluster family which are upregulated in patients with medulloblastoma, however they also note that to achieve further specificity, the crRNA should be modified.
Embedded Video 1: Animation of how the biosensor functions
All in all, the CRISPR-Cas system, whether it’s further polishing techniques using the Cas13a nuclease or another enzyme, holds a lot of promises for developing a detection platform to measure miRNA’s concentration at the point of care, which would made possible the early detection of a disease given that it’d compensate for the disadvantages of the previously mentioned methods such as microarrays, RNA-seq or qRT – PCR, which are restricted to be used in technical settings for research, lifting a barrier towards a wide-scale usage for health monitoring.
Causes of miRNA dysregulation
After sharing the above knowledge regarding miRNA deregulation and pathogenesis, interest might arise about why miRNA becomes dysregulated. To explain the identified causes of miRNA dysregulation, it’s first worth noting that miRNAs do not only regulate gene expression, but they themselves are also controlled by regulatory mechanisms, which may get altered during tumorigenesis, leading to either overexpression of oncomiRNAs or down-regulation of tumor suppressor miRNAs. As noted by (Marilena & Carlo, 2012), alterations in the level of miRNA expression can be caused by the following:
- Mutations that involve genes that transcribe into miRNAs can affect the expression of such miRNA. For example, in Chronic Lymphocytic Leukemia, mutations lead to the reduced expression of miR-15a and miR-16-1. Another example of an oncogenes affecting miRNA expression is represented by the oncoprotein MYC which is able to both induce oncogenic microRNAs,as the miR-17-92 cluster, and negatively regulate transcription of tumor suppressor miRNAs, such as let-7 and miR-29 family members
- Altered activity of transcription factors can also lead to miRNA’s abnormal concentrations. For example, an interesting regulatory loop has been demonstrated between ZEB1 transcription factor and miR-200 family: Epithelial-Mesenchymal Transitions (EMT) inducers ZEB1 and ZEB2 (both transcription factors) are direct target of miR-200 family members, and vice versa, ZEB1 has been shown to directly repress miR-200c and miR-141transcription.
- Altered miRNA’s concentration can also be due to epigenetic changes, such as altered DNA methylation, with DNA hypomethylation possibly leading to upmodulation of oncogenic miRNAs, while DNA hypermethylation leading to the silencing of miRNAs.
- Defects during miRNA biogenesis, such as for instance altered DROSHA or DICER activity. For example, it seems that the silencing of either DROSHA or DICER promotes tumorigenesis in vivo.
- miRNA’s expression level can also be affected by other miRNAs, thus creating a complex level of reciprocal interaction and regulation; for example, in a mouse model by Tang R and colleagues, miR-709 seem to be able to bind to pri-miR-15a/16-1 preventing its processing into pre-miR-15a/16-1 and thus leading to a suppression of miR-15a/16-1 maturation.
Figure 3 summarizes the mentioned causes of miRNA dysregulation.

Figure 3: Summary of the causes of miRNA dysregulation
Source: (Causes and Consequences of microRNA Dysregulation, 2012)by Marilena I. and Carlo C.
Challenges of miRNA diagnostics
After having discussed about miRNAs being potential biomarkers and detection platforms used to measure their concentration levels, it is also worth noting what are the challenges so far seen in this field of study. To begin, despite the high amount of research published pinpointing what miRNAs cause certain disease, there are cases where proposed miRNA biomarkers differ for different research groups even though the same disease is studied; as an example please examine (Ardekani & Naeini, 2010)and (Cheng, et al., 2015) where Alzheimer disease is analyzed; for the former, it’s mentioned that miR-9, miR-128a and miR-125b are upregulated in AD patients, while the latter identifies 15 upregulated miRNAs (hsa-miR-361-5p, hsa-miR-30e-5p, hsa-miR-93-5p, among others) and three downregulated miRNAs (hsa-miR-1306-5p, hsa-miR-342-3p and hsa-miR-15b-3p). Such incoherence can be explained due to differences in methodologies employed in the studies, given that in the end, there is not a standard protocol to research miRNAs, different research teams might perform differently miRNA sample collection or extraction, its processing and quality control, as well as later in the study the methods of analysis employed (Kioomars, Hamid, & Seyed, 2019).
Another challenge concerns the extraction of miRNA from a blood sample. As said before, miRNA from a blood sample may originate from extracellular vesicles called exosomes. Yet, there are investigations that probe whether enrichment of exosomes from biological samples are better than crude cell-free preparations for reliable biomarker measurements; and some reports suggest it may depend on the specific human disease. Vesicle purification can lower the RNA yield and integrity. Furthermore, some diseases may also alter exosome release and clearance, or administration of therapeutics can also alter blood volume, so in either case the miRNA measured can be skewed (Hanna, Hossain, & Kocerha, 2019).
Even with a standard set of steps that outlines how to isolate miRNAs from serum or sample, there are still a lot of knowledge that has not been sorted out. For instance, there is the challenge of choosing which miRNAs, out of the about 2000 miRNAs discovered, should be used as biomarker and for which diseases, given that there are some miRNAs, such as miR-21 which upregulation has been associated with colorectal, lung ,breast, prostate, liver, esophageal and endometrial cancers. There are also confounding factors that may influence how we establish its causal relationship with a disease, such factors can be age, gender, ethnicity, drugs or smoking, gut microbiome composition or diet; for example, what would be the real prognostic value of a certain or group of miRNAs for a childhood cancer instead of another age group’s cancer (Kioomars, Hamid, & Seyed, 2019). Also, it’s not very clear whether some miRNAs’ up or downregulation is a positive or negative sign of a disease’s development, as these miRNAs can both act as either tumor suppressors or tumorigenic. For example, let-7 microRNA can act as a tumor suppressor by suppressing growth rates through repression of the RAS pathway and sets of oncofetal genes, while at the same time, let-7 has oncogenic activity by promoting angiogenesis by repressing the activity of FIH1. (Sidi, et al., 2014 May 15)
Additionally, although miRNA promises a lot of diagnostic potential for the world’s most challenging diseases, this should not be confused with exploiting it as the sole predictor for pathogenesis. This is because phenotype is influenced by genotype and environmental factors, so all three variables should be taken into account in order to perform a disease diagnosis. With the intervention of artificial intelligence in medicine, next generation/deep sequencing should be taken as yet another tool available for the medical personnel to consult that’d aid in prognosticating a patient’s disease. This novel tool should be coupled with other definite testst and/or electronic health records that should be comprehensive more than ever, including information such as precise family history and genetic risk factors, environmental factors, or other X-RAY scans which information unable to be seen by the naked eye can now be seen by convolutional neural networks, among other diagnostic methods. Not only that, miRNAs are not the only circulating RNAs that should be the focus of researchers, as there yet a lot of non-coding RNAs yet to be discovered which could become even better biomarkers (Woolston, 2019) for the diverse diseases so far mentioned.
For medicine, countless opportunities are being opened for the future of medicine
Bibliography
Ardekani, A. M., & Naeini, M. M. (2010). The Role of MicroRNAs in Human Diseases . Review Article, Avicenna J Med Biotechx, 2(4): 161-179.
Celan, M., Rosignolo, F., Maggisano, V., Pecce, V., Iannone, M., Russo, D., & Bulotta, S. (2017). MicroRNAs as Biomarkers in Thyroid Carcinoma. Hindawi Review Article | International Journal of Genomics, Article ID 6496570.
Cheng, L., Doecke, J., R. S., Villemagne, V., Fowler, C., Rembach, A., . . . for the Australian Imaging, B. a. (2015). Prognostic serum miRNA biomarkers associated with Alzheimer disease shows concordance with neuropsychological and neuroimaging assessment. Molecular Psychiatry, 20, 1188–1196.
Courtney, W. (2017, April 19). O' REILLY Latest Articles. Retrieved August 11, 2020, from Digital detection: Large-scale ambition built on micro-scale fluidics: https://www.oreilly.com/content/digital-detection-large-scale-ambition-built-on-micro-scale-fluidics/
Dolores, S.-W., Judit, C.-S., Cristina, F., Isabel, H., Jordi, C., Alberto, L., . . . Alfredo, J. M. (2019). Altered microRNAs related to synaptic function as potential plasma biomarkers for Alzheimer's disease. Alzheimer's research & therapy, 11(1), 46.
Editors: Malik, Y., & Jens, A. (2014). miRNomics: MicroRNA Biology and Computational Analysis. Springer New York Heidelberg Dordrecht London.
Eric, T. (2019). Deep Medicine: How Artificial Intelligence Can Make Healthcare Human Again. New York: Hachette Book Group.
Esther, L. (2020, January 21). NATURE: TECHNOLOGY FEATURE . Retrieved August 09, 2020, from Technologies to watch in 2020: https://www.nature.com/articles/d41586-020-00114-4
Feng, Z., Yan, W., & Xiong, G. (2014). CRISPR/Cas9 for genome editing: progress,implications and challenges. Human Molecular Genetics, Vol. 23, Review Issue 1, R40 - R46.
Hammond, S. M. (2015). An overview of microRNAs. Advanced Drug Delivery Reviews, 87: 3–14. .
Hanna, J., Hossain, G., & Kocerha, J. (2019). The Potential for microRNA Therapeutics and Clinical Research. frontiers in Genetics, 10:478.
Hu, J., Xu, Y., Hao, J., Wang, S., Li, C., & Meng, S. (2012). MiR-122 in hepatic function and liver diseases. Protein & cell, 3(5), 364–371.
J, S., QH, W., & SC, Z. (2018). Role of microRNA-218-5p in the pathogenesis of chronic obstructive pulmonary disease. Eur Rev Med Pharmacol Sci., 22(13):4319-4324.
Jonathan, S. G., Omar O., A., Jeong, W. L., Patrick, E., Aaron, J. D., Julia, J., . . . Feng, Z. (2017). Nucleic acid detection with CRISPR-Cas13a/C2c2. HHS Public Access; Science, 356(6336): 438–442.
Kioomars, S., Hamid, R. K., & Seyed, H. G. (2019). Why have microRNA biomarkers not been translated from bench to clinic? Future Oncology, Vol. 15, No. 8.
Marilena, V. I., & Carlo, M. C. (2012). Causes and Consequences of microRNA Dysregulation. Cancer J , 18(3): 215–222.
Ming, Z., Hidetaka, H., Yifan, D., Lisha, M., David, K. C., Changyou, W., & Zhiming, C. (2016). Circulating Organ-Specific MicroRNAs Serve as Biomarkers in Organ-Specific Diseases: Implications for Organ Allo- and Xeno-Transplantation. International Journal of Molecular Sciences, 17(8): 1232.
Naveed, S., Tariq, H., Syed, Z., Ali, S., Antonio, P., Deming, Z., & Xiangmei, Z. (2018). miRNAs in Tuberculosis: NewAvenues for Diagnosisand Host-Directed Therapy. frontiers in Microbiology, 9:602.
Neo4j. (n.d.). Miroculus Case Study. Retrieved 08 11, 2020, from Machine Learning and Graph Technology Accelerate Medical Research: https://neo4j.com/case-studies/miroculus/
Ramkrishna, M., Clare, M. A., Jiang, W., Evan, G., & Christine, M. E. (2020). Pan-cancer analysis reveals cooperativity of bothstrands of microRNA that regulate tumorigenesisand patient survival. NATURE COMMUNICATIONS, Journal Article 1 - 15.
Richard, B., Julia, B., Claire, C., Mailin, M., Sibylle, M., Wilfried, W., . . . Gerald, A. U. (2019). CRISPR/Cas13a-Powered Electrochemical Microfluidic Biosensor for Nucleic Acid Amplification-Free miRNA Diagnostics. Advanced Materials, 31, 1905311.
Sathipati, S. Y., & Ho, S.-Y. (2018). Identifying a miRNA signature for predicting the stage of breast cancer. SCIENTIFIC REPORTS, 8:16138.
Sharda, T., & Kalpana, G. (2015). miR-122 is a Unique Molecule with Great Potential in Diagnosis, Prognosis of Liver Disease, and Therapy Both as miRNA Mimic and Antimir. Curr Gene Ther. , 15(2): 142–150. .
Sidi, C., Yuan, X., Wu, X., Le, C., Arjun, B., Eric, L. B., . . . Phillip, A. S. (2014 May 15). Global microRNA depletion suppresses tumor angiogenesis. Genes & Development, 28(10): 1054–1067.
Woolston, C. (2019, October 16). How RNA therapies could be used to tackle the world's biggest killer. Retrieved August 14, 2020, from nature OUTLOOK: https://www.nature.com/articles/d41586-019-03073-7
Yuanyue, S., Xiaoming, Z., Ru, H., & Da, X. (2019). High-Fidelity and Rapid Quantification of miRNA Combining crRNAProgrammability and CRISPR/Cas13atrans-Cleavage Activity. Analytical Chemistry, 91, 5278−5285.
Zhi, L., Jianwei, W., Yuyu, X., Mengchen, G., Kai, M., Rui, X., . . . Xingyin, L. (2020). Implications of the virus-encoded miRNA and host miRNA in the pathogenicity of SARS-CoV-2.
Comentarios